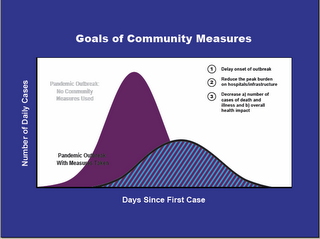
Flatten The Curve - CDC circa Feb. 1st 2007
#15,225
Up until a few months ago, few Americans knew what `flatten the curve' meant, although it has been part and parcel of our nations pandemic preparedness plan since early in 2007 (see The CDC Does NPI). Now, it is part of everyone's vocabulary.
The theory behind it is based on the disparate experiences of St. Louis, which implemented strict social distancing during the 1918 Spanish Flu - and Philadelphia - which did not (see Why NPIs Will Be Our 1st Line Of Defense Against COVID-19).But until a couple of months ago - at least in modern times - it hadn't been tested under genuine pandemic conditions. And no one really knew if it was practical, or that it would work.
Even today, with ample evidence that yes, you can `flatten the curve' - even with a highly contagious respiratory virus - there are unanswered questions about the optimum level of social distancing, the required duration, and how to safely emerge from `lockdown' without reigniting a viral firestorm.As nations try to come out of their economically, and socially, painful mitigation measures we'll begin to get a glimpse of what works, and what doesn't. While politics and mounting economic pressures will undoubtedly play a role in how, and when they act, most nations will be using a variety of computer models and simulations to guide them.
As we discussed a little over a week ago, in CDC: COVID-19 Forecasting, computer models are often quite useful, but they can't be taken literally. What you get out of them depends primarily on what data you put into them.And in the study below, four different age-based social distancing scenarios are used over a 6-week period in a medium sized American or European City (based on Seattle, WA demographics), and run 1,000 times while varying 3 parameters; the R0, the latent period, and duration of infectiousness.
The study is lengthy, complex, and will be tough sledding for those (like myself) who are statistically challenged. But I've selected some of the less technical passages as excerpts below. As always, you are encouraged to follow the link and read the full report.
Volume 26, Number 8—August 2020
Research
Evaluating the Effectiveness of Social Distancing Interventions to Delay or Flatten the Epidemic Curve of Coronavirus Disease
Abstract
By April 2, 2020, >1 million persons worldwide were infected with severe acute respiratory syndrome coronavirus 2. We used a mathematical model to investigate the effectiveness of social distancing interventions in a mid-sized city. Interventions reduced contacts of adults >60 years of age, adults 20–59 years of age, and children < 19 years of age for 6 weeks.
Our results suggest interventions started earlier in the epidemic delay the epidemic curve and interventions started later flatten the epidemic curve.
We noted that, while social distancing interventions were in place, 20% of new cases and most hospitalizations and deaths were averted, even with modest reductions in contact among adults.
However, when interventions ended, the epidemic rebounded. Our models suggest that social distancing can provide crucial time to increase healthcare capacity but must occur in conjunction with testing and contact tracing of all suspected cases to mitigate virus transmission.
(SNIP)
Discussion
The term “flatten the curve,” originating from the Centers for Disease Control and Prevention (26), has been used widely to describe the effects of social distancing interventions. Our results highlight how the timing of social distancing interventions can affect the epidemic curve. In our model, interventions put in place and lifted early in the epidemic only delayed the epidemic and did not flatten the epidemic curve.
When an intervention was put in place later, we noted a flattening of the epidemic curve. Our results showed that the effectiveness of the intervention will depend on the ratio of susceptible, infected, and recovered persons in the population at the beginning of the intervention.
Therefore, an accurate estimate of the number of current and recovered cases is crucial for evaluating possible interventions. As of April 2, 2020, the United States had performed 3,825 tests for SARS-CoV-2 per 1 million population (27). By comparison, Italy had performed 9,829 tests/1 million population (27). Expanding testing capabilities in all affected countries is critical to slowing and controlling the pandemic.
Some evidence suggests that persons who recover from COVID-19 will develop immunity to SARS-CoV-2 (28). However, at this point the duration of immunity is unclear. If immunity lasts longer than the outbreak, then waning immunity will not affect the dynamics of the epidemic. Furthermore, persons who recover from COVID-19 could re-enter the workforce and help care for the most vulnerable groups. However, if immunity is short-lived, for instance on the order of weeks, persons who recover could become re-infected, and extensions to social distancing interventions might be necessary.(SNIP)
Our results must be interpreted with caution. Hospitalizations and deaths averted during the first 100 days in our model would likely occur later if the interventions are lifted without taking any further action, such as widespread testing, self-isolation of infected persons, and contact tracing. As in any model, our assumptions could overestimate the effect of the interventions. However, quantifying the short-term effects of an intervention is vital to help decision makers estimate the immediate number of resources needed and plan for future interventions.
(SNIP)
Taken together, our results suggest that more aggressive approaches should be taken to mitigate the transmission of SARS-CoV-2. Social distancing interventions need to occur in tandem with testing and contact tracing to minimize the burden of COVID-19. New information about the epidemiologic characteristics of SARS-CoV-2 continues to arise. Incorporating such information into mathematical models such as ours is key to providing public health officials with the best tools to make decisions in uncertain times.
Dr. Matrajt is a research associate at the Fred Hutchinson Cancer Research Center. Her research interests include using quantitative tools to understand infectious disease dynamics and to optimize public health interventions.
Dr. Leung is a postdoctoral research fellow at the Fred Hutchinson Cancer Research Center. Her research interests include using mathematics to understand infectious disease transmission.
The original idea behind using NPIs to `flatten the curve' was to extend the length of a pandemic wave by lowering its daily peak, thereby reducing the crushing impact on hospitals and other community resources.
While there was certainly hope, there was no guarantee that the actual number of infections, or the direct loss of life from the virus, would be any less. The pain would just be spread out over a longer period of time.It was expected that collateral damage would be lessened, and that would save many lives. And if a vaccine, or an effective therapeutic could be developed before the pandemic had run its course, that could save additional lives as well.
Suddenly we are faced with an unexpected dilemma. Strict lockdowns - while they have exacted a terrible economic cost - have worked in reducing infections and deaths far more effectively than anyone imagined.It is likely that hundreds of thousands - and perhaps millions - of lives have been spared by the incredible sacrifices that societies have made around the world in the past four months. We have almost certainly avoided the collapse of numerous healthcare systems as well.
The $64 question is, what do we do now?
How do we ease out of lockdown and revive the economies without destroying our unexpected early success? As the above study cautions, gains made because of social distancing could be lost if interventions are lifted without taking further actions.
While models and computer simulations may not be able to give us all of the answers, by feeding in new scenarios and fresh data each day, they can hopefully help tell us `where there be dragons, or shoals ahead' as we navigate through these uncharted waters in the months ahead.
None of us, however, should expect it to be smooth sailing.